What about the monetization of data? The rising flood of data offers promising potential for companies - experts in the mobility industry are largely agreed on this. However, it is also becoming apparent that these potentials have not yet been seized for the most part as of today. Against this background, this article from the Detecon study 'Data Strategies in Smart Mobility' highlights opportunities and challenges for monetizing data. An insight into the evaluation of data is also given in more detail and the prerequisites for the monetization of data are shown.
Data in the mobility industry - crucial for the future but unused?
"Data is the new oil" - This thesis met with majority approval in interviews with experts in the mobility industry within the framework of the Detecon study Data Strategies in Smart Mobility (not yet published). And this is also quite understandable (see article Smart Mobility - Data Strategies in the Mobility of the Future). In the midst of an industry shaped by digitization, traditional mobility providers are facing increasingly fierce competition, including now emerging mobility startups as well as tech giants once removed from the industry.
The fact that change is also being taken seriously by traditional players can be seen not least in the anchoring of the future fields of Electric, Autonomous, Connected and Shared/Services - in the corporate strategies of Daimler (CASE) and BMW (ACES). For some of these future fields, data as well as its collection, use and exchange across company boundaries are immanently important. In the article 'Data in the haystack', it was already shown that data is being collected on a massive scale according to the principle of Big Data, but that as of today, few holistic approaches to the use or monetization of data have been established. This fact is also confirmed by a current cross-industry study by BARC. The results of the study show that only 17% of the experts surveyed state that they have implemented a solution for the actual monetization of data and only 12% are currently launching concrete pilot projects. The reasons for this can be manifold.
In this study article, specific options for monetizing data are presented in greater detail and the main challenges in each case are outlined. In addition, a concrete model is presented, which can serve as a reference point for the strategic evaluation and positioning of data in the company.
Concrete opportunities and key challenges for monetizing data
When dealing with the topic of data, it quickly becomes clear that there is no single golden road by which one can monetize one's data. The value of data depends to a large extent on the specific context in which it can develop its potential.
An established way to use data is in business intelligence (BI) systems. BI allows data to be collected, evaluated and presented in a structured manner in the context of the company (see Lecture Notes in Business Information Processing). As a result, business decisions can be supported in a multi-layered and data-based manner - for example, the optimization of business processes through production flow data. Although this does not result in a direct monetization of data in companies, it does result in an indirect monetization.
In expert interviews, reference was often made in this regard to the highly efficient and data-driven production processes within the framework of Industrie 4.0. If one dares to look beyond this, however, it becomes clear that companies often do not currently have a uniform data strategy and that data islands are the rule. Especially for the targeted evaluation of customer data from multiple channels - also called customer touchpoints - the lack of a central customer ID represents a major hurdle for BI. After all, how can the behavior of customers be understood on the basis of data if each channel separately only depicts a section? Concrete efforts to establish integrated data collection and evaluation through data pools and big data analytics can be observed in the mobility industry, but such an undertaking is by no means trivial. Companies need competencies for this, and tech companies are currently shining with them.
With data, the portfolio of products and services can be continuously expanded, improved and also increasingly interlinked. One key to this is so-called data-driven development: the consideration of data collection by products already during their development.
While integrated data evaluation is also important here, the handling of customer data must be viewed particularly critically for the establishment of cross-company mobility ecosystems. In Germany in particular, there are strong concerns about data protection. More than 62% of Germans are concerned about the sovereignty of their data, according to a study by Symantec . The willingness of German customers to share personal data with companies and thus also to use data-driven products and services is low by international comparison. This is compounded for companies by the fact that the legal effectiveness of the EU Regulation DSGVO since 2018, the data processing of personal data is regulated. Customers often lack the right incentive system that would encourage data sharing. After all, why share your personal data without directly benefiting from it?
At this point, it is worth making the leap to another way of monetizing data - establishing entirely new business models. These are innovative products and services to take the lead in the data-driven future. This opportunity to monetize data is understandably seen by mobility experts as highly relevant but also particularly challenging.
The following examples illustrate the diverse potential for innovation. A new data-driven business model can be found in the telecommunications industry. Motionlogic (a subsidiary of Telekom) sells analyses of traffic and movement flows based on anonymous signaling data from the mobile network. This is made possible by the smart linking and analysis of data generated from the core business - mobile communications. Another example comes from the insurance industry: In the Pay as you Drive (PAYD) business model, customers are encouraged to release data on their driving behavior to insurance companies in return for a corresponding payment. Using this data, insurance companies can make more accurate risk assessments, whereupon insurance costs are reduced if the driving style is appropriate - a win-win situation in the sense of an incentive system. Platforms for the (cross-industry) exchange and trade of data continue to establish themselves more and more. BMW, for example, provides anonymized vehicle telematics data for business customers through its BMW CarData data platform - but only with the customer's consent. Also worth mentioning in this context is Otonomo, an up-and-coming startup from Israel that enables traditional mobility providers such as Daimler to monetize data directly via their neutral data platform (see Business Insider for more on this).
A few more examples of innovative and data-driven business models can be cited - but if you take a look at the industry, it also becomes clear that the full potential for data-driven innovation is far from exhausted. Long-term market constellations have yet to emerge. In addition to several other challenges, traditional players in particular often still lack a fundamental basis for driving the monetization of data: The establishment of a strategic position for data in the company and the associated understanding of which data is particularly valuable in the individual company context.
Click here to download the German-language discussion paper
Smart Mobility – Daten und Data Sharing als Enabler für smarte Ökosysteme
Mark HeinrichThe value of data depends on its individual context. Companies can gain an initial indication of this using the four dimensions VRIO.
VRIO for strategic evaluation and positioning of data in the company
The VRIO framework developed by Jay Barney describes a well-founded possibility for the strategic evaluation of an organization's resources. If data is viewed as an organizational resource, this framework can be used to make initial assessments of which data are particularly valuable and how they need to be positioned in the company in order to develop their value.
VRIO covers the four dimensions of Value, Rareness, Imitability and Organization. The better data performs in these dimensions, the higher its competitive relevance and thus its value for the organization. This can be illustrated as follows: A data set is particularly valuable if it opens up opportunities for the organization (Value) that are not available to the competition (Rareness) and cannot be easily imitated (Imitability). To exploit these unique opportunities, the organization must also be able to use the data set effectively (Organization). Thus, a suitable organizational framework for monetizing data must be created.
Opportunities to monetize data in terms of the Value dimension are many and varied. Data can deliver great added value for companies both directly and indirectly; opportunities for this - from supporting decisions to creating new business models - have already been outlined above. The important thing here is to ensure data quality. Incomplete, incorrect or outdated data sets can not only be worthless, but can also actively cause damage if used improperly, for example through serious errors in business processes. Furthermore, it can be assumed that data becomes more valuable when it is related to each other. For example, more business opportunities can be derived from an overall understanding of customer behavior across a complete customer journey than from data on a single touchpoint.
Putting the dimension of rareness in the context of data in a smart mobility ecosystem is at first glance a contradiction, because certain data must be shared between players to enable a seamless mobility experience and thus cannot be rare. However, this only applies to certain categories of data, such as real-time data or mobility master data (see article Data Sharing in the Mobility-as-a-Service Age). For other data (such as insights into customer behavior), which offer attractive opportunities and are not available to every competitor to the same extent, it is quite understandable that they should be kept under wraps at all costs.
If data is also difficult to imitate by the competition (imitability), it is truly a strategically valuable resource. The greater the effort required by the competition to obtain the data sets, the better for the company itself. For example, building your own connected car fleet to collect telematics data would require a great deal of effort, whereas a single customer survey would be comparatively simple.While all three dimensions together give an indication of how valuable data is for the individual company context, the company must also have the ability to make a profit from it (organization). Building such capabilities requires the strategic positioning of data within the company and the establishment of a suitable operating model.
Mark HeinrichOnly by creating the right framework can companies realize the full potential for monetizing data.
In order to establish and sustainably anchor the Operating Model, it is advisable to draw on proven practices and methods of organizational transformation. The optimal approach for such an undertaking depends to a large extent on the individual company context and goes beyond the content of this article. An initial overview of important fields of action is provided by the diagram below, which visualizes the VRIO framework with four central dimensions for setting up and anchoring a suitable operating model.
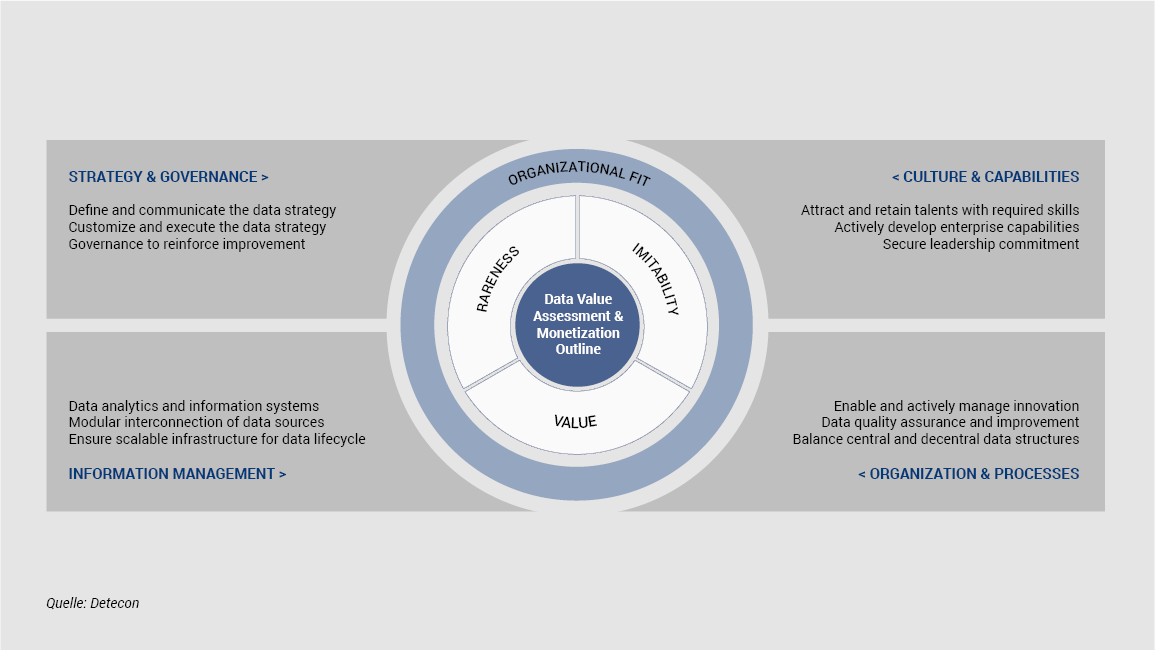
Although the results of the Detecon study 'Data Strategies in Smart Mobility' show that potentials for the monetization of data in the mobility industry have often not yet been seized, there is also a clear confidence among the mobility providers surveyed to shape their data-driven future independently. Opportunities to leverage and monetize data are many and varied. VRIO offers an initial starting point for identifying valuable data and embedding it in a suitable organizational context.